Using deep neural networks to understand the temporal structure of human memory
Navez, Lucie
Promotor(s) :
Louppe, Gilles
;
D'Argembeau, Arnaud
Date of defense : 26-Jun-2023/27-Jun-2023 • Permalink : http://hdl.handle.net/2268.2/17788
Details
Title : | Using deep neural networks to understand the temporal structure of human memory |
Author : | Navez, Lucie ![]() |
Date of defense : | 26-Jun-2023/27-Jun-2023 |
Advisor(s) : | Louppe, Gilles ![]() D'Argembeau, Arnaud ![]() |
Committee's member(s) : | Drion, Guillaume ![]() Franci, Alessio ![]() |
Language : | English |
Discipline(s) : | Engineering, computing & technology > Computer science |
Institution(s) : | Université de Liège, Liège, Belgique |
Degree: | Master en ingénieur civil en informatique, à finalité spécialisée en "intelligent systems" |
Faculty: | Master thesis of the Faculté des Sciences appliquées |
Abstract
[en] This thesis explores the temporal structure of human memory through the use of deep neural networks. The research aims to extend the study conducted by Roseboom et al. in "Activity in perceptual classification networks as a basis for human subjective time perception", which investigates the process of time perception based on non-temporal factors, by using a vision classification deep neural network in order to mimic human vision. Building upon this work, the present study focuses on predicting recalling times of episodic memories using a different dataset and further explores the influence of various neural network architectures as well as identifying critical layers and other factors in the prediction process.
In addition to the extension of the work of Roseboom et al. to a new task, this study also extends the research scope by investigating the influence of different neural network architectures and identifying the layers that play crucial roles in accurate recall time predictions. Through a deep analysis, it becomes obvious that the temporal dynamics of time perception in humans can be effectively transposed to the specific task of episodic memories recall. Furthermore, we discover that certain types of neural network architectures, particularly more recent ones, such as change detection networks, exhibit superior performance in capturing and modeling the temporal dynamics of memory when compared to traditional feedforward neural networks.
This research provides an analysis of using deep neural networks to comprehend the temporal structure of human memory. The findings have significant implications for the fields of cognitive science and neural networks. Future research in this area can exploit these insights to further advance our understanding of the temporal aspects of memory and contribute to the progress of knowledge in the field.
File(s)
Document(s)
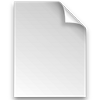

Description: Full text
Size: 9.13 MB
Format: Adobe PDF
Cite this master thesis
The University of Liège does not guarantee the scientific quality of these students' works or the accuracy of all the information they contain.