Football Game Events Detection Through Features Extraction and Deep Learning
Castin, Martin
Promoteur(s) :
Van Droogenbroeck, Marc
Date de soutenance : 25-jui-2018/26-jui-2018 • URL permanente : http://hdl.handle.net/2268.2/4536
Détails
Titre : | Football Game Events Detection Through Features Extraction and Deep Learning |
Titre traduit : | [fr] Détection d'événements dans un match de football à l'aide d'extraction de caractéristiques et d'apprentissage profond |
Auteur : | Castin, Martin ![]() |
Date de soutenance : | 25-jui-2018/26-jui-2018 |
Promoteur(s) : | Van Droogenbroeck, Marc ![]() |
Membre(s) du jury : | Embrechts, Jean-Jacques ![]() Wehenkel, Louis ![]() Barnich, Olivier |
Langue : | Anglais |
Nombre de pages : | 73 |
Mots-clés : | [en] Deep Learning [en] Computer Vision [en] Machine Learning [en] tracking [en] classification [en] sequential |
Discipline(s) : | Ingénierie, informatique & technologie > Sciences informatiques |
Institution(s) : | Université de Liège, Liège, Belgique |
Diplôme : | Master en ingénieur civil en informatique, à finalité spécialisée en "intelligent systems" |
Faculté : | Mémoires de la Faculté des Sciences appliquées |
Résumé
[en] Reducing production costs is a key asset for the future of broadcasting companies. An automatic understanding of Football matches could help to alleviate these costs by helping the production operators. Part of this understanding for instance consists in the ability to automatically determine if a video sequence contains an interesting event as a shot or a goal or not.
This problem is tackled in a bottom-up way, features are first extracted to be fed in a Machine Learning model to detect events. These features are chosen according to what humans intuitively use for the same task: the players positions and poses. Using very recent Deep Learning techniques as well as traditional tracking approaches, the players positions are extracted from the images and tracked in a real-world frame.
Various deep neural networks are then tested against these extracted features, as well as ground truth tracking data, to determine whether possible flaws are coming from the feature extraction algorithm.
The developed algorithm reaches about 80% accuracy when using players positions. It was found, however, that best results (about 90 % accuracy) were obtained when using the ball track, only available in the ground truth data, and recent temporal convolution techniques.
Fichier(s)
Document(s)
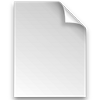

Description:
Taille: 111.99 kB
Format: Adobe PDF
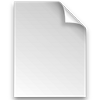

Description:
Taille: 24.6 MB
Format: Adobe PDF
Annexe(s)
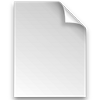

Description:
Taille: 27.37 MB
Format: Unknown
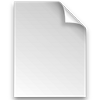

Description:
Taille: 38.08 MB
Format: Unknown
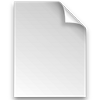

Description:
Taille: 37.46 MB
Format: Unknown
Citer ce mémoire
L'Université de Liège ne garantit pas la qualité scientifique de ces travaux d'étudiants ni l'exactitude de l'ensemble des informations qu'ils contiennent.