Machine learning and computer vision for automatic analysis of figure skating videos
Capouillez, Anaïs
Promotor(s) :
Geurts, Pierre
Date of defense : 6-Sep-2018/7-Sep-2018 • Permalink : http://hdl.handle.net/2268.2/5431
Details
Title : | Machine learning and computer vision for automatic analysis of figure skating videos |
Author : | Capouillez, Anaïs ![]() |
Date of defense : | 6-Sep-2018/7-Sep-2018 |
Advisor(s) : | Geurts, Pierre ![]() |
Committee's member(s) : | Marée, Raphaël ![]() Wehenkel, Louis ![]() Van Droogenbroeck, Marc ![]() |
Language : | English |
Discipline(s) : | Engineering, computing & technology > Computer science |
Institution(s) : | Université de Liège, Liège, Belgique |
Degree: | Master en sciences informatiques, à finalité spécialisée en "intelligent systems" |
Faculty: | Master thesis of the Faculté des Sciences appliquées |
Abstract
[en] During competitions, skaters perform many elements that the majority of viewers cannot differentiate. With a classifier of elements, it would be easier for them to recognize elements. The aim of this master thesis was to analyze figure skating videos to classify elements performed by skaters. The classification of figure skating elements was conducted on a dataset of elements performed by skaters. This dataset was created for this master thesis. Each element was annotated with body parts coordinates, that were detected with the Windows Portable demo of OpenPose (see https://github.com/CMU-Perceptual-Computing-Lab/openpose). Then, the skater was tracked among detected people with a custom tracker. Two datasets corresponding to the same data were created. A dataset with features corresponding to body parts coordinates, and another dataset with features corresponding to measurements made from these body parts coordinates. Elements were then classified with the k-nearest neighbors classifier. Finally, performances were assessed. Using the two different types of features, only some elements were very well classified. Therefore a combination of both types of features was implemented to use the strengths of both types of features. With this combination, all elements were very well classified, except one element (upright spins), for which only half of samples were correctly classified. Results are encouraging, especially considering the limited size of the dataset.
File(s)
Document(s)
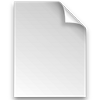

Description: -
Size: 7.14 MB
Format: Adobe PDF
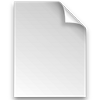

Description: -
Size: 127.91 kB
Format: Adobe PDF
Annexe(s)
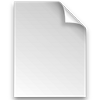

Description: -
Size: 10.78 kB
Format: Unknown
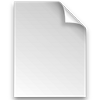

Description: -
Size: 44.56 kB
Format: Adobe PDF
Cite this master thesis
The University of Liège does not guarantee the scientific quality of these students' works or the accuracy of all the information they contain.